Synthetic Data
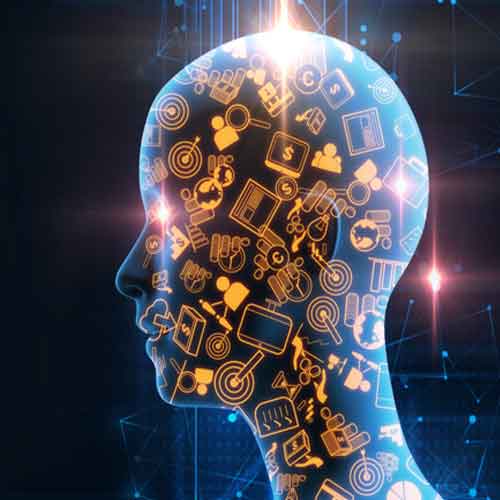
Data is the lifeblood of modern artificial intelligence. Getting the right data is both an important and challenging part of building powerful AI. Collecting quality data from the real world is complicated, expensive and time-consuming. This is where synthetic data comes in. Synthetic data can be defined as information which is manufactured artificially and not obtained by direct measurement. Synthetic data can help improve AI.
Synthetic data may closely resemble real-world data, but and however, engineers need to be extremely cautious when using such data as this data, at the end of the day, is not real.
If you’re constantly improving, engaging with your target audience, and boosting your brand’s look and expectations, you’re on the right track. However, this is only the start of getting your business to truly stand out. To do that, you'll need something more. You'll need to use synthetic data.
Synthetic data is redefining the business world. Using synthesized data makes it significantly easier to understand your client base, gather necessary intel, produce solid AI programs, and deliver a fantastic repertoire to consumers. It’s also relatively easy to set up and use.
The significant advantage of this is that the original text is already digitally known, which means that the AI’s output can be compared to what was computed originally. Thus, a closed-loop system can improve the ability of the AI to recognise handwriting while never needing to see real-world examples of handwriting.
There are several examples, creating a climate model using current data that doesn’t support the current consensus can be altered so that it does, and a climate AI learning from this model would be fed potentially false information.
Another example of manipulated models could be the creation of simulated social media data with large amounts of political biases and stereotypes that don’t conform to real-world data.
Training an AI to learn from this would result in an AI that can only see the world through the eyes of the manipulated model, and this could be dangerous if deployed in a real-world scenario such as account monitoring, flagging, and automated bans.
Simply generating data from nothing is not going to be representative of the real world and will therefore result in an algorithm that most likely can’t function when it encounters real data.
The solution is to collect a subset of data, analyze and identify trends and ranges in it, and then use this data to generate a large pool of random data that very likely represents how the data would look if we collected all of it ourselves.
This is where the value of synthetic data truly lies. No longer do we have to run around tirelessly collecting data which then needs to be cleaned and processed before use. However, there are several different ways you can get started with synthetic learning, especially in the data, sales, and marketing sectors.
See What’s Next in Tech With the Fast Forward Newsletter
Tweets From @varindiamag
Nothing to see here - yet
When they Tweet, their Tweets will show up here.