NLP & GenAI continue to complement each other
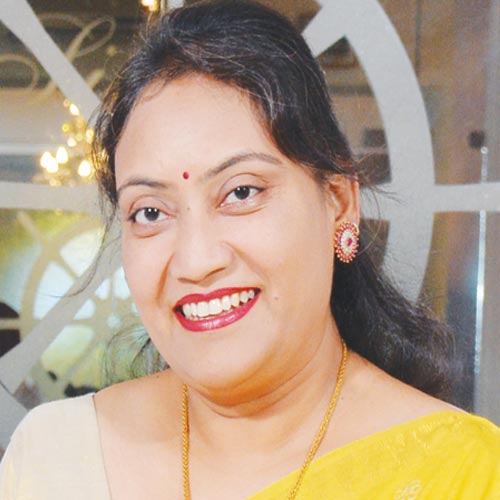
Artificial intelligence (AI) is rapidly transforming industries and businesses of all sizes. While AI holds immense potential to improve efficiency, productivity, and decision-making, adopting and implementing AI effectively can be challenging.
Companies across all industries are recognizing the immense potential of artificial intelligence to transform their operations, enhance customer experiences, and gain a competitive edge.
Despite widespread focus on generative AI throughout the current year, the percentage of respondents who said they had introduced or were planning to introduce tools that used natural language processing in the coming year ticked up from 67% in 2023 to 70% in 2024, according to the survey.
The increasing adoption of natural language processing tools despite the widespread focus on generative AI in 2023 highlights the complementary nature of these two technologies. NLP provides a foundation for understanding and processing human language, while GenAI can utilize this understanding to generate new text, translate languages, and create other forms of creative content.
The rise of NLP adoption suggests that organizations are recognizing the value of NLP in automating tasks, improving customer interactions, and gaining insights from large volumes of text data. NLP tools can be used for tasks such as sentiment analysis, chatbots, and summarizing documents.
While GenAI is grabbing headlines due to its ability to produce creative and human-like text, NLP remains an essential component for many organizations. NLP provides the underlying understanding of language that allows GenAI to function effectively.
The coexistence of NLP and GenAI is analogous to the relationship between a chef and a kitchen appliance. The question arises whether transformer-based architecture can surpass in-house human-written code depends on the specific task and the quality of the human-written code.
In general, transformer-based models have shown impressive performance in a variety of natural language processing tasks, such as machine translation, text summarization, and question answering. However, human-written code can often be more flexible and adaptable than transformer-based models, especially when dealing with complex or nuanced tasks.
Despite these challenges, one of the biggest bottlenecks in technology is the availability of human resources for coding. In many cases, there is intellectual work with high added value, but many of the software development activities are relatively simple and should be automated soon.
See What’s Next in Tech With the Fast Forward Newsletter
Tweets From @varindiamag
Nothing to see here - yet
When they Tweet, their Tweets will show up here.