How IoT can address modern day challenges
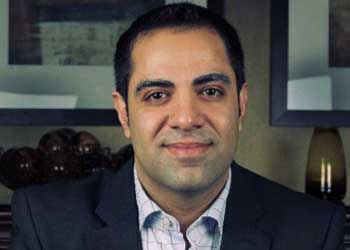
We all have personally experienced the opportunities that connectivity on-the-go brings along through the mobile phones we carry. Mobile phones have revolutionised the way we all travel, purchase, pay and connect with critical services like education and healthcare. Beyond mobile phones connecting the humans, the next big wave of technology is “Internet of Things (IoT)” that involves connecting different physical aspects of the world around us to the cyber world. We already see many connected devices being launched from home appliances to health monitoring bands and vehicle tracking.
IoT promises to connect more than a trillion devices in the coming decade. Like how even the most optimistic vision for ubiquity of mobile phones and its advantages, done a decade ago, has been surpassed, one can only imagine the new opportunities and applications connectivity of trillions of devices will open. Opportunities will emerge across all the three major domains – consumer (such as home automation), enterprise (such as marrying of online-offline retail and smart buildings) and industrial (largely around industrial automation also commonly viewed as Industry 4.0). While each of these will have their own unique challenges that must be addressed, there are three common themes, starting from data collection to data analysis and eventually taking decisions based on the analysis, that are to be catered to across each one of them.
When it comes to data collection, it is important to consider and support reliable “brown-field” connectivity. It will be prohibitively expensive to replace all the existing unconnected (or dumb) devices with connected (or smart) devices. So, one needs to develop solutions for brown-field connectivity i.e. figuring out low cost ways to connect existing dumb devices with internet and enabling data collection around their performance. Such a connectivity is also challenged by poor infrastructure, especially in emerging economies like India. These include intermittent network connectivity and unreliable and poor quality power. Considering such challenges, the IoT systems must include reliable and sophisticated edge computing. In other words, analyse the information and perform as much decision making offline as possible and only rely on online connectivity to update the learned models for iteratively improved decision making.
Once reliable systems are put in place for data collection, one needs to start understanding the utility of collected data and providing real value to the customer. With cheap connectivity and low cost sensors available, collecting millions and billions of data points, once the first problem around connectivity is addressed, is an easy task. Its however difficult to find the needle in this haystack i.e. infer useful information, for intelligent decision making, from all this collected data. While the world talks about problem of “big data”, I would rather argue that the problem is indeed of “useful data” even if it is much smaller in volume. At the end of the day, the customer will not pay for the data but for the utility derived from the data. It is important to both figure out the right applications that can deliver “real value” to the end customer and ensure that the collected data is sufficient and complete for performing required analysis to deliver that value proposition. This will likely require multiple iterations both in terms of finding the right product-market fit as well as to figure out the right sensory information to increasingly deliver value to identified product-market fit.
Finally, it is important to close-the-loop. Rugged data collection and sophisticated analysis should result in “automated and intelligent” actions that eventually deliver the value. All too often, the analysis is delivered in the form of complex charts and plots leaving the decision making and final action in the hands of people. There are multiple problems in such an approach. Firstly, you are increasing the work for the people on the ground and no one likes their work to be increased. Secondly, such a feedback is then expecting people to change their behaviour and perform as per the provided feedback. How often do we really change behaviour based on other people around us? How can we expect people to change behaviour based on the input provided by machines? Correspondingly, systems should be put in place to automatically deliver the output of the intelligent analysis. As an example, if it’s around optimisation of power consumption of air conditioner, the system should not give feedback to the user on what to do and when. Instead the system should automatically control the air conditioner in an intelligent way to optimise the comfort of the user and the energy consumption of the air conditioner.
IoT is an enabler. The technologies involved allow us to collect plethora of data from systems which were otherwise offline (and hence les understood) so far. Building the right tools for reliable and useful data collection, together with machine learning models for inferring the necessary decision making and eventually delivering this analysis in an automated manner will provide end customers with an end-to-end system that makes their existing operations more efficient and deliver value thereof. We have only scratched the tip of the iceberg. It is beyond anyone’s imagination what such ubiquitous data collection can enable in the next couple of decades.
Nikhil Rajpal
CEO, Hero Electronix
See What’s Next in Tech With the Fast Forward Newsletter
Tweets From @varindiamag
Nothing to see here - yet
When they Tweet, their Tweets will show up here.