Deep Learning has the capability to process both Structured and Unstructured data
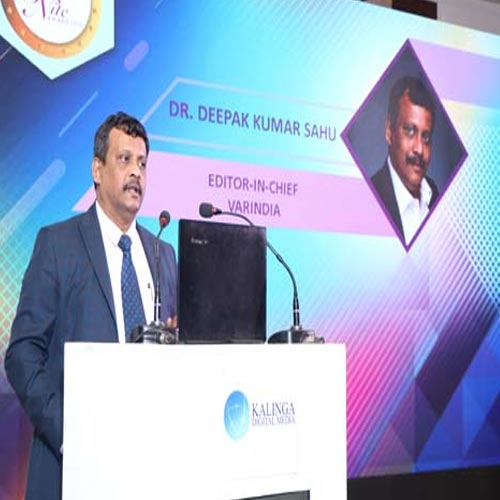
Deep learning, when applied to data science, can offer better and more effective processing models. Its ability to learn unsupervised drives continuous improvement in accuracy and outcomes. Deep learning, a computer program trains to conduct classification algorithms from text, images or sound. It also offers data scientists with more reliable and concise analysis results. 80% of the world’s data is unstructured. Much of your company’s data may be unstructured. In order to get the most mileage out of AI and machine learning, it helps to have your data formatted.
There may be methods of incorporating AI and machine learning into your ongoing operations that you’re not yet aware of. That’s why it’s important to format as much of your data as you can within a database. This helps you get ahead of the curve. Deep Learning is called Deep because of the number of additional “Layers” we add to learn from the data. One of the key reasons deep learning is more powerful than classical machine learning is that it creates transferable solutions. Deep learning algorithms are able to create transferable solutions through neural networks: that is, layers of neurons/units.
It is important to acknowledge here that the definition of deep learning itself has been evolving as it embraces developments within the larger fold of AI. Gary Marcus, a pioneer in deep learning, has stated four possibilities for the future of deep learning, namely, unsupervised learning that would enable systems to determine their own objectives and do problem solving, integration of deep learning with symbolic systems that would make inferences more accurate, developing learning models through better insights from cognitive and developmental psychology and AI becoming multidimensional to deal with the complexities of the problem.
According to Yann LeCun, Chief AI Scientist at Facebook, the next phase of AI will not be supervised but reinforced. He has elaborated on self- supervised learning, i.e. the idea of learning to represent the world before learning to perform a specific task. As per Nikko Strom, a scientist, integrating symbolic reasoning and learning efficiently from interactions with the world are two major challenges that deep learning should be addressing. It is anticipated that deep learning systems of the future would be able to handle non-uniform data available in multiple formats. There are also exciting interdisciplinary initiatives underway that could have a significant impact on deep learning.
See What’s Next in Tech With the Fast Forward Newsletter
Tweets From @varindiamag
Nothing to see here - yet
When they Tweet, their Tweets will show up here.